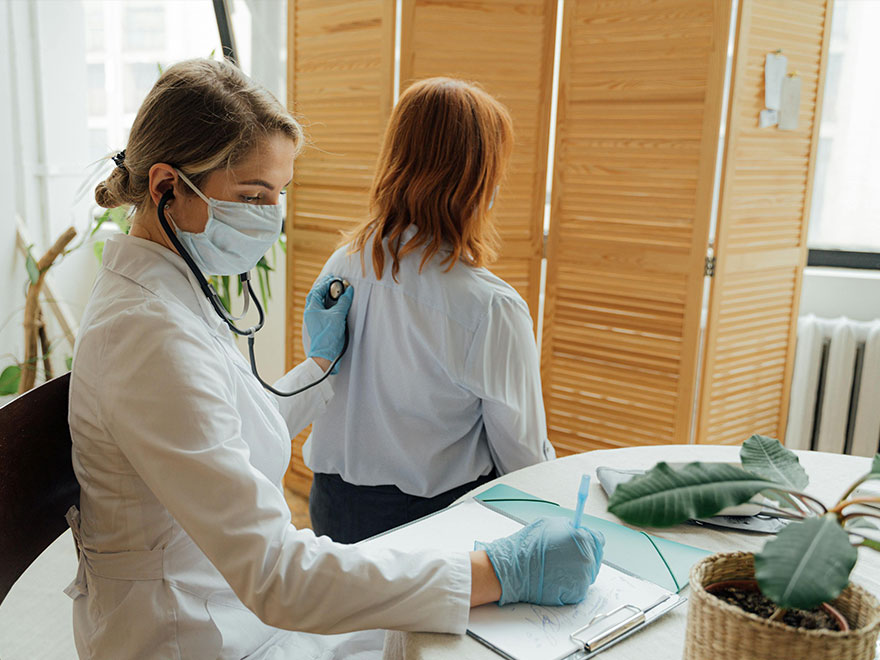
Jae-Kwon Kim
Abstract
The efficient scheduling of resources within emergency departments (EDs) is crucial to minimizing patient length of stay (LoS) times and maximizing the utilization of limited resources. Reducing patient wait times can enhance the operation of emergency departments and improve patient satisfaction and the quality of medical care. This study develops a simulation model using Discrete Event Simulation (DES) methodology, examining six resource scheduling policies that consider different combinations of general and senior physicians. By leveraging six scheduling policies and machine learning techniques, this model dynamically identifies the most effective scheduling policy, based on a comprehensive dataset of ED visits in South Korea. The ED simulation achieves an accuracy rate of 90% and demonstrates that our proposed integrated machine learning approach reduces average length of stay (LoS) to approximately 322.91 min, compared to 327.10 min under traditional methods. This study underscores the potential of integrating DES and machine learning to enhance resource management in EDs.
Keywords:
emergency department; resource scheduling; simulation; machine learning
reference: